Advancements in Autonomous Vehicles Through Deep Learning
Exploring Deep Learning's Impact on Future Autonomous Vehicles
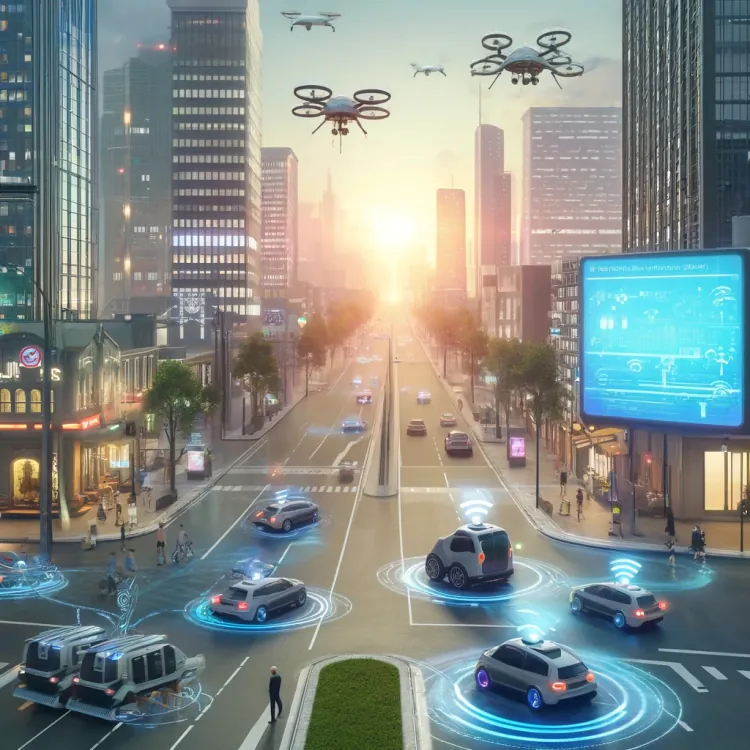
Introduction
Autonomous vehicles (AVs) represent a groundbreaking shift in transportation, driven by deep learning technologies. This article explores the current state of AVs, the role of deep learning, and what future advancements we might expect.
Understanding Deep Learning and Its Role in Autonomous Vehicles
Deep learning, a subset of artificial intelligence, mimics the human brain's ability to learn from large amounts of data. This capability is crucial for autonomous vehicles, enabling them to recognize patterns, make decisions, and adapt to new scenarios without human input.
Key Advancements in Autonomous Vehicles Enabled by Deep Learning
Deep learning has facilitated several key advancements in autonomous vehicle technology:
- Enhanced Sensory Perception and Object Recognition: These systems enable vehicles to accurately identify and classify objects in diverse environments.
- Improved Decision-Making Algorithms: Deep learning algorithms help vehicles make informed decisions in real-time, enhancing road safety.
- Advancements in Predictive Analytics: Vehicles can anticipate potential hazards and adjust their driving accordingly.
- Adaptive Learning Systems: AVs continuously learn and adapt, improving their functionality over time.
Case Studies of Deep Learning Success in Autonomous Vehicles
Companies like Tesla and Waymo are at the forefront of integrating deep learning into their AVs. These technologies have not only improved safety but have also set new standards for vehicle autonomy.
Challenges and Limitations of Deep Learning in Autonomous Vehicles
Despite its potential, deep learning faces challenges such as data privacy concerns, ethical dilemmas in decision-making, and high computational demands.
Future Prospects: What’s Next for Deep Learning in Autonomous Vehicles?
The future of AVs looks promising with advancements in AI technology. Regulatory frameworks and continuous technological improvements will likely pave the way for more widespread adoption of AVs.
Conclusion
Deep learning is revolutionizing autonomous vehicles, offering significant improvements in safety and efficiency. As this technology advances, it will continue to shape the future of transportation.
FAQs
- What is deep learning and why is it important for autonomous vehicles? Deep learning processes complex data to improve vehicle autonomy and safety.
- How do autonomous vehicles use deep learning to improve safety? By enhancing object recognition and decision-making, deep learning increases safety.
- What are the main challenges facing the integration of deep learning in autonomous vehicles? Key challenges include ethical decision-making, data privacy, and computational requirements.
This article provides a comprehensive look at how deep learning is set to transform autonomous driving, promising a future of safer, more efficient transportation solutions.
What's Your Reaction?
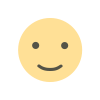
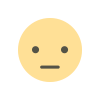
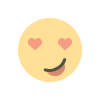
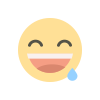
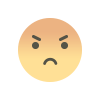
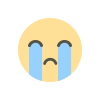
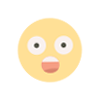